www.ptreview.co.uk
12
'23
Written on Modified on
NEW MLCOMMONS RESULTS HIGHLIGHT IMPRESSIVE COMPETITIVE AI GAINS FOR INTEL
Habana Gaudi2 and 4th Gen Intel Xeon Scalable processors deliver leading performance and optimal cost savings for AI training.
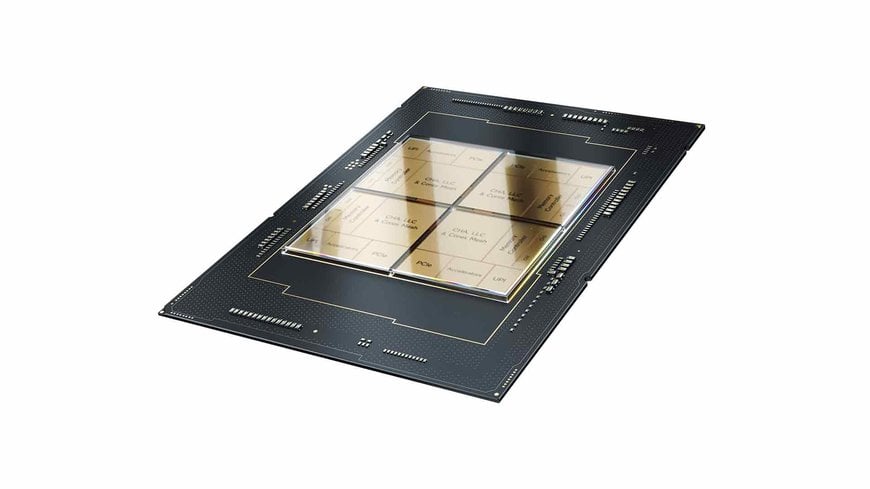
4th Gen Intel® Xeon® Scalable processor, code-named Sapphire Rapids. (Credit: Intel Corporation)
MLCommons published results of its industry AI performance benchmark, MLPerf Training 3.0, in which both the Habana® Gaudi®2 deep learning accelerator and the 4th Gen Intel® Xeon® Scalable processor delivered impressive training results.
Why it Matters
The current industry narrative is that generative AI and large language models (LLMs) can run only on Nvidia GPUs. New data shows that Intel’s portfolio of AI solutions provides competitive and compelling options for customers looking to break free from closed ecosystems that limit efficiency and scale.
The latest MLPerf Training 3.0 results underscore the performance of Intel’s products on an array of deep learning models. The maturity of Gaudi2-based software and systems for training was demonstrated at scale on the large language model, GPT-3. Gaudi2 is one of only two semiconductor solutions to submit performance results to the benchmark for LLM training of GPT-3.
Gaudi2 also provides substantially competitive cost advantages to customers, both in server and system costs. The accelerator’s MLPerf-validated performance on GPT-3, computer vision and natural language models, plus upcoming software advances make Gaudi2 an extremely compelling price/performance alternative to Nvidia’s H100.
On the CPU front, the deep learning training performance of 4th Gen Xeon processors with Intel AI engines demonstrated that customers can build with Xeon-based servers a single universal AI system for data pre-processing, model training and deployment to deliver the right combination of AI performance, efficiency, accuracy and scalability.
About the Habana Gaudi2 Results
Training generative AI and large language models requires clusters of servers to meet massive compute requirements at scale. These MLPerf results provide tangible validation of Habana Gaudi2’s outstanding performance and efficient scalability on the most demanding model tested, the 175 billion parameter GPT-3.
Results Highlights:
MLCommons published results of its industry AI performance benchmark, MLPerf Training 3.0, in which both the Habana® Gaudi®2 deep learning accelerator and the 4th Gen Intel® Xeon® Scalable processor delivered impressive training results.
Why it Matters
The current industry narrative is that generative AI and large language models (LLMs) can run only on Nvidia GPUs. New data shows that Intel’s portfolio of AI solutions provides competitive and compelling options for customers looking to break free from closed ecosystems that limit efficiency and scale.
The latest MLPerf Training 3.0 results underscore the performance of Intel’s products on an array of deep learning models. The maturity of Gaudi2-based software and systems for training was demonstrated at scale on the large language model, GPT-3. Gaudi2 is one of only two semiconductor solutions to submit performance results to the benchmark for LLM training of GPT-3.
Gaudi2 also provides substantially competitive cost advantages to customers, both in server and system costs. The accelerator’s MLPerf-validated performance on GPT-3, computer vision and natural language models, plus upcoming software advances make Gaudi2 an extremely compelling price/performance alternative to Nvidia’s H100.
On the CPU front, the deep learning training performance of 4th Gen Xeon processors with Intel AI engines demonstrated that customers can build with Xeon-based servers a single universal AI system for data pre-processing, model training and deployment to deliver the right combination of AI performance, efficiency, accuracy and scalability.
About the Habana Gaudi2 Results
Training generative AI and large language models requires clusters of servers to meet massive compute requirements at scale. These MLPerf results provide tangible validation of Habana Gaudi2’s outstanding performance and efficient scalability on the most demanding model tested, the 175 billion parameter GPT-3.
Results Highlights:
- Gaudi2 delivered impressive time-to-train on GPT-31: 311 minutes on 384 accelerators.
- Near-linear 95% scaling from 256 to 384 accelerators on GPT-3 model.
- Excellent training results on computer vision — ResNet-50 8 accelerators and Unet3D 8 accelerators — and natural language processing models — BERT 8 and 64 accelerators.
- Performance increases of 10% and 4%, respectively, for BERT and ResNet models as compared to the November submission, evidence of growing Gaudi2 software maturity.
- Gaudi2 results were submitted “out of the box,” meaning customers can achieve comparable performance results when implementing Gaudi2 on-premise or in the cloud.
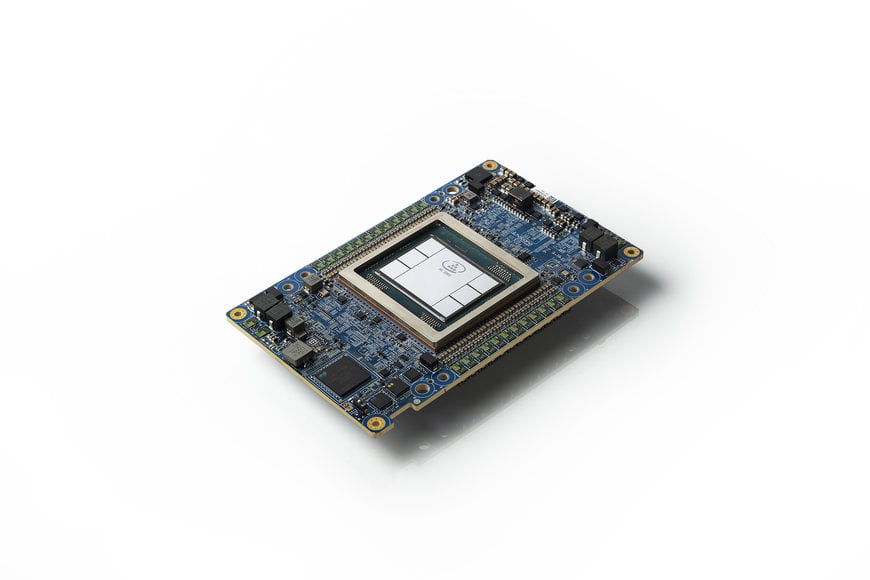
Habana Gaudi2 Mezzanine Card (Credit: Habana Labs)
About Gaudi2 Software Maturity
Software support for the Gaudi platform continues to mature and keep pace with the growing number of generative AI and LLMs in popular demand.
- Gaudi2’s GPT-3 submission was based on PyTorch and employed the popular DeepSpeed optimization library (part of Microsoft AI at scale), rather than custom software. DeepSpeed enables support of 3D parallelism (Data, Tensor, Pipeline) concurrently, further optimizing scaling performance efficiency on LLMs.
- Gaudi2 results on the 3.0 benchmark were submitted in the BF16 data type. A significant leap in Gaudi2 performance is expected when software support for FP8 and new features are released in 2023’s third quarter.
About the 4th Gen Xeon Processors Results
As the lone CPU submission among numerous alternative solutions, MLPerf results prove that Intel Xeon processors provide enterprises with out-of-the-box capabilities to deploy AI on general-purpose systems and avoid the cost and complexity of introducing dedicated AI systems.
For a small number of customers who intermittently train large models from scratch, they can use general-purpose CPUs, and often on the Intel-based servers they are already deploying to run their businesses. However, most will use pre-trained models and fine-tune them with their own smaller curated data sets. Intel previously released results demonstrating that this fine-tuning can be accomplished in only minutes using Intel AI software and standard industry open-source software.
MLPerf Results Highlights:
As the lone CPU submission among numerous alternative solutions, MLPerf results prove that Intel Xeon processors provide enterprises with out-of-the-box capabilities to deploy AI on general-purpose systems and avoid the cost and complexity of introducing dedicated AI systems.
For a small number of customers who intermittently train large models from scratch, they can use general-purpose CPUs, and often on the Intel-based servers they are already deploying to run their businesses. However, most will use pre-trained models and fine-tune them with their own smaller curated data sets. Intel previously released results demonstrating that this fine-tuning can be accomplished in only minutes using Intel AI software and standard industry open-source software.
MLPerf Results Highlights:
- In the closed division, 4th Gen Xeons could train BERT and ResNet-50 models in less than 50 mins. (47.93 mins.) and less than 90 mins. (88.17 mins.), respectively.
- With BERT in the open division, the results show that Xeon was able to train the model in about 30 minutes (31.06 mins.) when scaling out to 16 nodes.
- For the larger RetinaNet model, Xeon was able to achieve a time of 232 mins. on 16 nodes, allowing customers the flexibility of using off-peak Xeon cycles to train their models over the course of a morning, over lunch or overnight.
- 4th Gen Xeon with Intel® Advanced Matrix Extensions (Intel® AMX) delivers significant out-of-box performance improvements that span multiple frameworks, end-to-end data science tools and a broad ecosystem of smart solutions.
MLPerf, generally regarded as the most reputable benchmark for AI performance, enables fair and repeatable performance comparison across solutions. Additionally, Intel has surpassed the 100-submission milestone and remains the only vendor to submit public CPU results with industry-standard deep-learning ecosystem software.
These results also highlight the excellent scaling efficiency possible using cost-effective and readily available Intel Ethernet 800 Series network adapters that utilize the open source Intel® Ethernet Fabric Suite Software that’s based on Intel oneAPI.
More Context: Performance Metrics Based on MLPerf v3.0 Training (Benchmark Results) | MLCommons Announcement
These results also highlight the excellent scaling efficiency possible using cost-effective and readily available Intel Ethernet 800 Series network adapters that utilize the open source Intel® Ethernet Fabric Suite Software that’s based on Intel oneAPI.
More Context: Performance Metrics Based on MLPerf v3.0 Training (Benchmark Results) | MLCommons Announcement