www.ptreview.co.uk
21
'24
Written on Modified on
TDK News
SIMPLIFYING MACHINE LEARNING FOR EVERYONE: COLLABORATION OF SENSORS AND SOFTWARE
TDK's streamlined software solution has the potential to significantly accelerate innovation in manufacturing and expand into rapidly evolving fields like Edge AI and Industry 4.0.
www.tdk.com
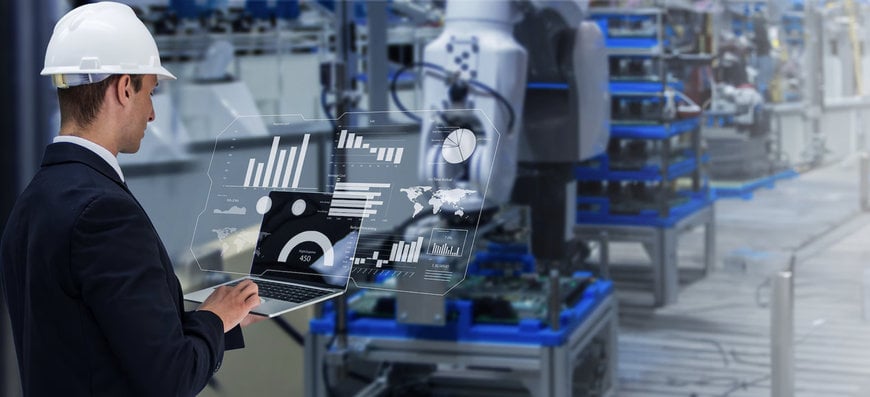
With the growing popularity of applications such as generative AI, the demand for machine learning has surged. Typically, implementing machine learning involves using sophisticated software and complex algorithms to process large datasets. TDK offers a streamlined software solution that enables the efficient application of machine learning to a wide range of sensor data. This solution has the potential to significantly accelerate innovation in manufacturing and expand into rapidly evolving fields like Edge AI and Industry 4.0.
How an automated sorting robot accurately differentiates containers
Doosan Robotics and TDK SensEI have collaborated to develop an AI-powered robot capable of accurately recognizing and sorting various containers, including bottles, cans, and paper cups. Honored as a CES 2024 Innovation Awards recipient in the Artificial Intelligence category, this robot uses Edge AI embedded in its software to identify different container types. Through the processing of current and torque sensor data in real time, the robot recognizes objects to allow for precise identification. The trained edge AI model leverages these features to ensure each container is sorted correctly.
AI sorting robot developed by Doosan Robotics and TDK
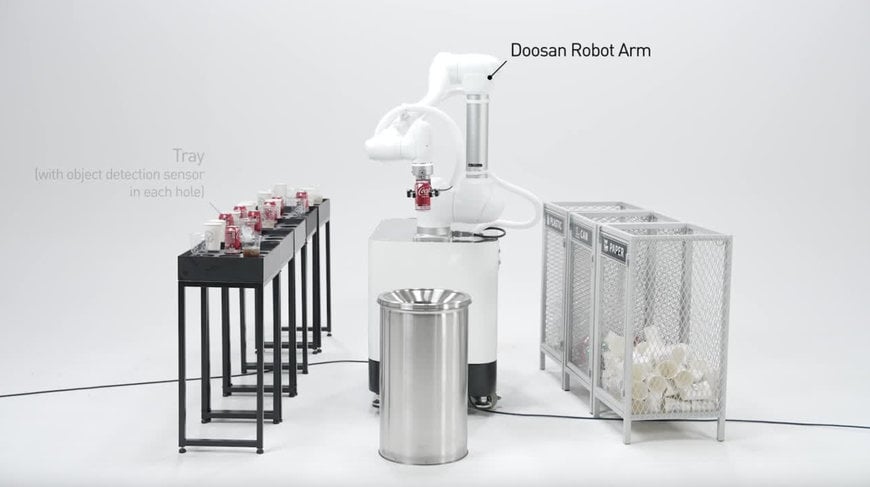
The AI robot precisely recognizes the container’s colour, shape, size, etc., and sorts it appropriately.
The ever-expanding potential of machine learning
Machine learning, which trains computers to identify patterns from vast amounts of data, has become widely applied in areas such as healthcare, manufacturing, and financial services. Generative AI, which is a hot topic today, is also rooted in machine learning. As the Internet of Things (IoT) continues to grow, machine learning has become a crucial tool for utilizing data gathered from sensors.
Machine learning can be divided into three primary methodologies:
- Reinforcement learning: A process where systems learn optimal actions by ingesting enormous amounts of data, improving through trial and error.
- Supervised learning: A technique where data input, along with correct examples, is used to make predictions on new data.
- Unsupervised learning: A method that automatically uncovers patterns and structures in unlabeled data.
The choice of method depends on the nature of the data and the desired outcomes. However, machine learning requires advanced and complex software to analyze patterns in large datasets, making its implementation both time-intensive and costly.
Machine learning categorization
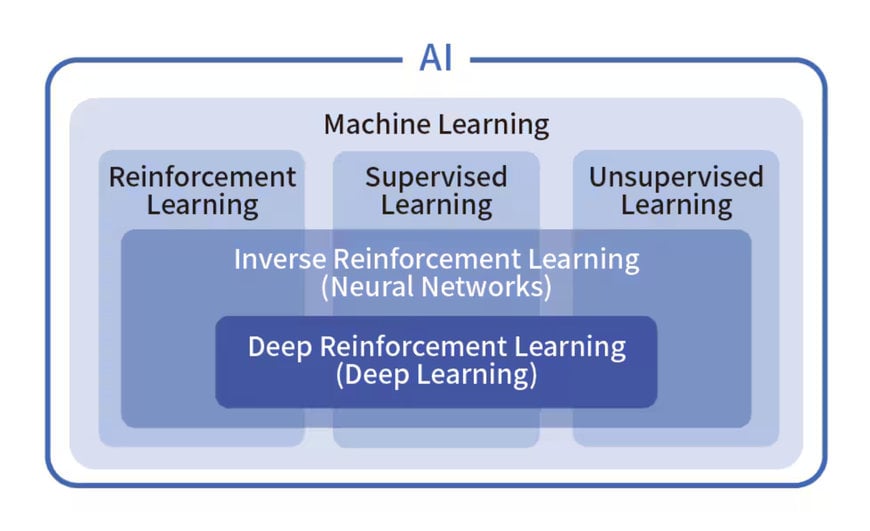
Machine learning falls into various categories, such as reinforcement learning, supervised learning, and unsupervised learning.
Software that makes machine learning implementation a breeze
TDK SensEI's AutoML is a software platform designed to simplify the implementation of complex machine learning systems for all users. With its intuitive, web-based interface, users can easily complete the steps required to build a machine learning model, including data collection, preprocessing, and training. Paired with TDK’s wide range of sensor products, AutoML offers powerful solutions for condition-based monitoring (CbM) by combining motion sensors with machine learning. This enables the detection of abnormalities in industrial machinery, helping to prevent serious faults before they occur.
In a typical AutoML CbM system, motion sensors continuously track the operational conditions of motors and other machinery components, while AutoML processes the sensor data in real-time. When deviations from normal operating patterns are detected, AutoML flags these anomalies, allowing for the early prediction of potential failures. This enables more precise maintenance scheduling, preventing breakdowns before they happen. By using this solution, machine uptime can be significantly increased compared to traditional maintenance approaches that address issues only after serious failures occur.
Edge AI motor monitoring demonstration
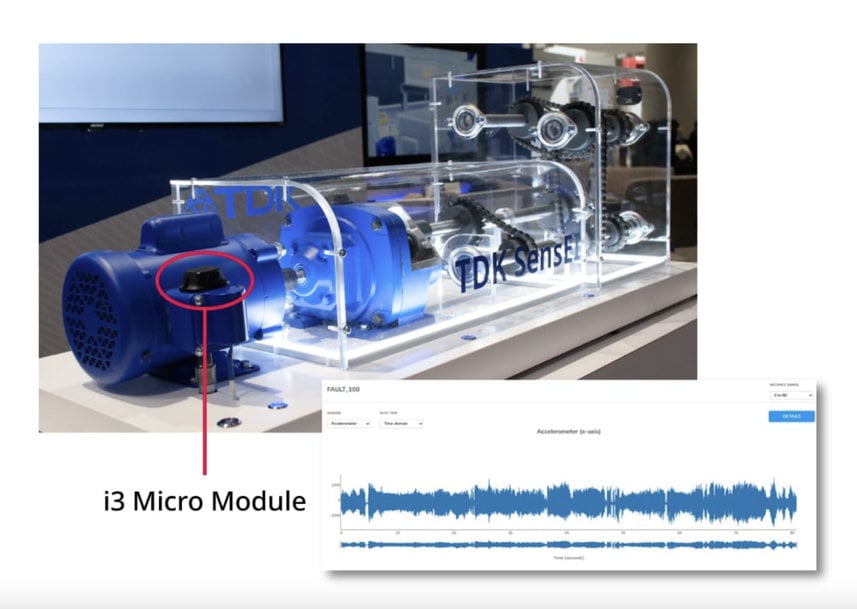
In this demonstration by TDK SensEI, data from a sensor attached to an electric motor was used to train the system, enabling it to continuously monitor and differentiate three states: Idle, Normal, and Fault.
As the potential for advanced data utilization continues to grow, this technology is expected to be deployed across a wide range of industries, including manufacturing, transportation infrastructure, building maintenance, healthcare, energy, agriculture, logistics, automotive, retail, and smart cities. Its applications are set to transform operations in numerous sectors by improving efficiency, enhancing predictive capabilities, and driving innovation.
The expanding applications of edge AI
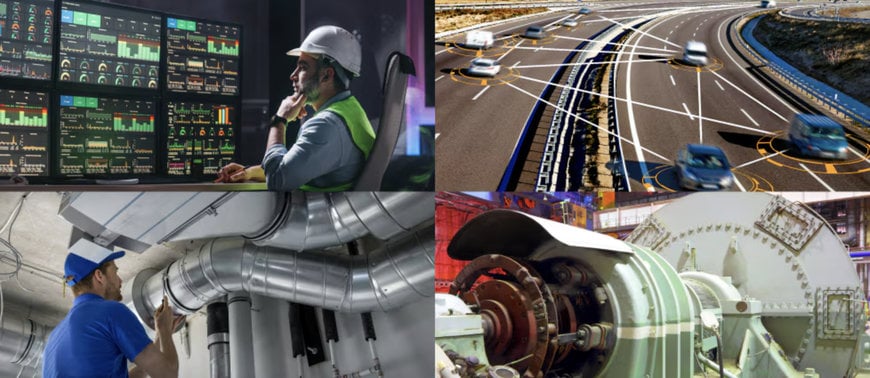
As opportunities for sensor data utilization grow, the technology is expected to expand into various fields, including optimizing production lines in manufacturing, improving transportation infrastructure, optimizing building energy usage, and predictive maintenance of equipment.
Machine learning categorization
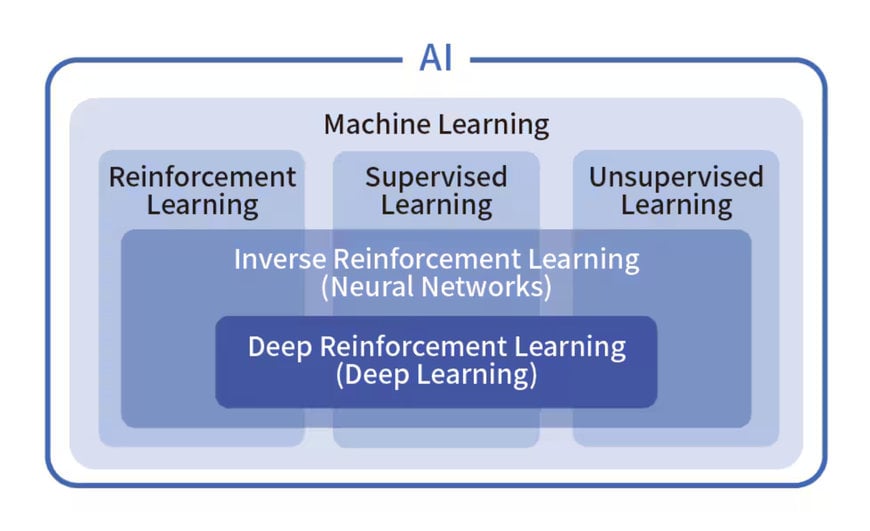
Machine learning falls into various categories, such as reinforcement learning, supervised learning, and unsupervised learning.
Software that makes machine learning implementation a breeze
TDK SensEI's AutoML is a software platform designed to simplify the implementation of complex machine learning systems for all users. With its intuitive, web-based interface, users can easily complete the steps required to build a machine learning model, including data collection, preprocessing, and training. Paired with TDK’s wide range of sensor products, AutoML offers powerful solutions for condition-based monitoring (CbM) by combining motion sensors with machine learning. This enables the detection of abnormalities in industrial machinery, helping to prevent serious faults before they occur.
In a typical AutoML CbM system, motion sensors continuously track the operational conditions of motors and other machinery components, while AutoML processes the sensor data in real-time. When deviations from normal operating patterns are detected, AutoML flags these anomalies, allowing for the early prediction of potential failures. This enables more precise maintenance scheduling, preventing breakdowns before they happen. By using this solution, machine uptime can be significantly increased compared to traditional maintenance approaches that address issues only after serious failures occur.
Edge AI motor monitoring demonstration
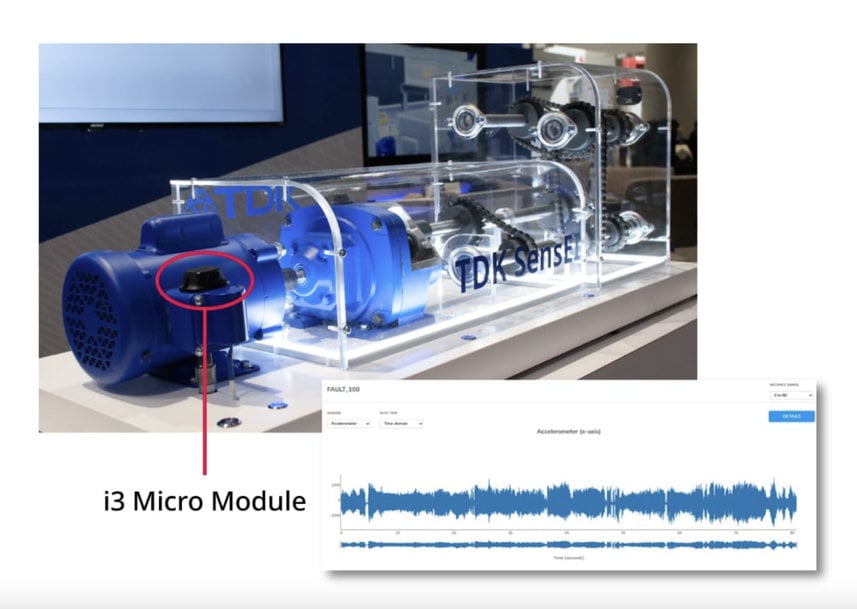
In this demonstration by TDK SensEI, data from a sensor attached to an electric motor was used to train the system, enabling it to continuously monitor and differentiate three states: Idle, Normal, and Fault.
As the potential for advanced data utilization continues to grow, this technology is expected to be deployed across a wide range of industries, including manufacturing, transportation infrastructure, building maintenance, healthcare, energy, agriculture, logistics, automotive, retail, and smart cities. Its applications are set to transform operations in numerous sectors by improving efficiency, enhancing predictive capabilities, and driving innovation.
The expanding applications of edge AI
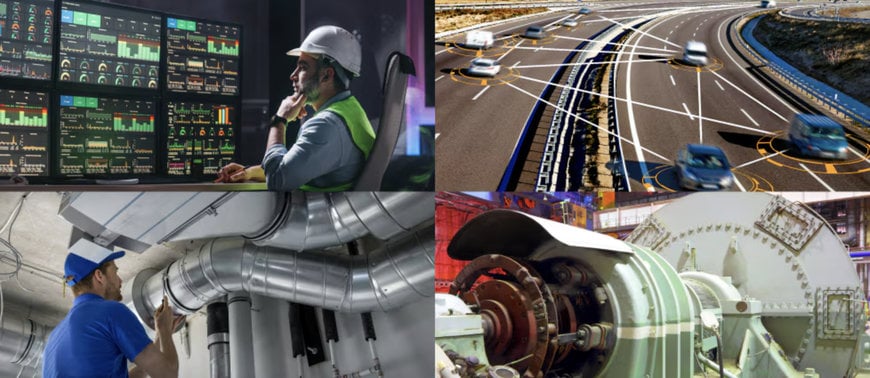
As opportunities for sensor data utilization grow, the technology is expected to expand into various fields, including optimizing production lines in manufacturing, improving transportation infrastructure, optimizing building energy usage, and predictive maintenance of equipment.