www.ptreview.co.uk
23
'22
Written on Modified on
Stemmer imaging News
Software solutions for machine vision based on deep learning
MVTec is one of STEMMER IMAGING’s main partners in the development of artificial vision software solutions. On the occasion of the release of HALCON 22.11, the newest version of MVTec’s comprehensive standard software for machine vision, we spoke to Chady Elahmad, Regional Sales Manager NW Europe, about machine vision developments such as deep learning or big data and our strong partnership.
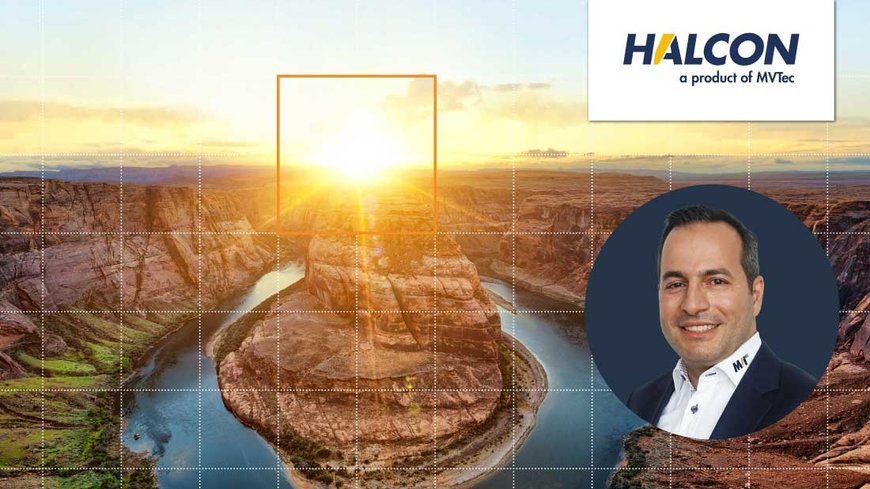
MVTec software products offer a range of functions and methods based on technologies such as deep learning. What are the advantages of image processing with deep learning?
In our understanding, deep learning is a subset of machine learning in which artificial neural networks are being used. In the traditional approach, the exact solution part must explicitly be described step by step to be able to extract the image properties. The difference with deep learning is that the neural networks are trained on large image datasets which can be used to identify and evaluate the relevant image properties.
Thus, this approach can master previously unsolvable problems. However, deep learning remains a complex technology; what we do at MVTec is that we focus on industrial machine vision and do research to integrate deep learning technologies, such as classification anomaly detection, deep OCR, object detection, semantic segmentation and so on, very easy to use and integrate with our software.
What role does big data play in the boom of deep learning and its machine vision applications?
When we speak of deep learning, we always speak of data, so by nature, deep learning requires a big number of images for the training and evaluation of the neural networks. This data must be collected and organized before the actual development of application can begin. It means that customers must have the infrastructure to acquire and organize such a big amount of data.
What we are seeing at the moment is that very often, when customers want to use deep learning, they don’t have enough images available. Especially of defects, it is always a challenge to have images. To help in such a situation, MVTec developed a technology that's named anomaly detection, available in MVTec HALCON as well as MVTec MERLIC, which allows to train a network with only 20 to 50 images of good samples.
Can you give us some application examples?
Deep Learning is a good solution when working with natural products, such as fruits or plants because the product will never be the same, but always very similar. In other applications, for example in the metal industry (detection of defects, scratches, scuffs, etc.), inspection without deep learning achieves 95 % accuracy, while with deep learning accuracy levels of 99 % are achieved.
The battery production sector is a good example of this. We worked with a customer who was looking at how to reduce the production cost and thus decided to go with a machine vision approach.
Depending on the type of defects, they had samples of only 10 or up to 100 images available, not sufficient to solve with classical methods. Instead, they used MVTec’s anomaly detection, available in our software products.
They could achieve 100% detection rate on the application and even found defect errors that were not clearly defined ahead of time because the concept of anomaly detection is that you are providing images of good samples and it would detect anything that deviates from the status “good”.
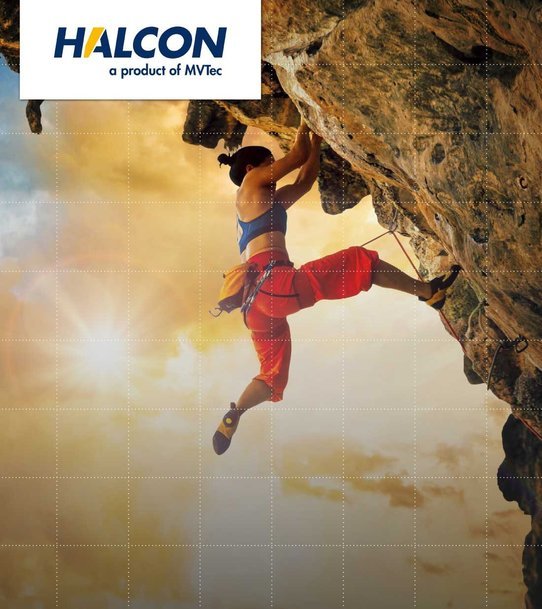
The new version MVTec HALCON 22.11 contains many new and improved features. Which industries do you think will benefit most from these features?
Both HALCON and MERLIC are standard products, so they are not targeted at specific sectors. But let’s take a close look at some of HALCON’s new features:
There is, for example, the 3D Gripping Point Detection, which can be used to robustly detect surfaces on any object that is suitable for gripping with suction. As this is a CAD-less approach, which eliminates the need to train object surfaces, customers can build their bin-picking applications much more flexible.
HALCON 22.11 also introduces a new data type called “memory block”, which can be used to store and transfer binary data in HALCON as well as further process it with other applications. This increases the software's compatibility with machine communication protocols (e.g., OPC UA).
Furthermore, there is the option to encrypt all data that can be serialized. In this way, trained deep learning models can now also be protected – an important step to protect our customers’ expertise and investment which has gone into collecting the data and training the models.
Another new feature helps customers to better understand the deep learning black box, as it increases the traceability of corresponding processes. The Guided GradCam supplies even more precise clues, in the form of a heat map, as to which regions of the image are relevant for the decision made by the deep learning network. For example, this enables users to investigate misclassifications.
www.stemmer-imaging.com