www.ptreview.co.uk
20
'20
Written on Modified on
EUAutomation News
Adapting to the new normal
Manufacturers must accelerate digital transformation to survive the coronavirus pandemic.
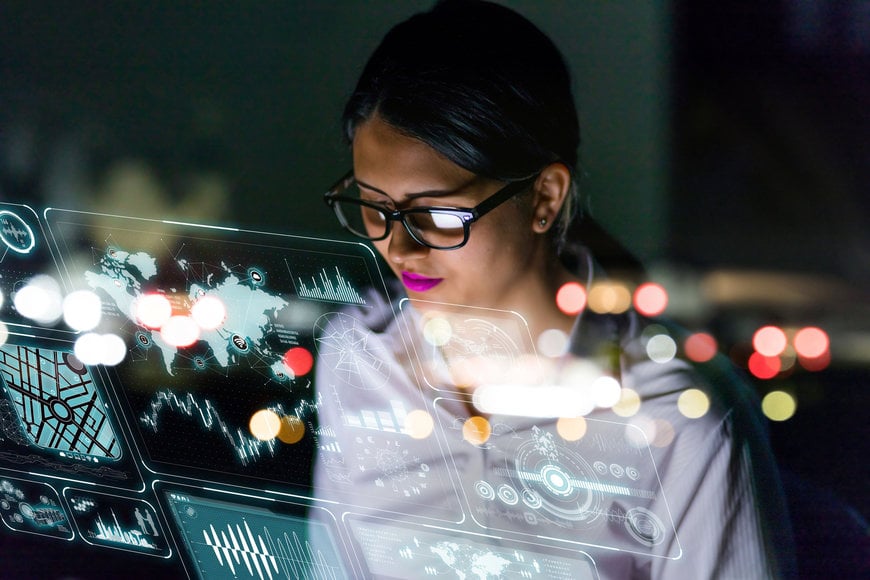
COVID-19 is disrupting business on an unprecedented scale. From reduced workforces to implementing social distancing measures and work from home policies, the pandemic has redefined normal business practice. Here, Claudia Jarrett, country manager at obsolete parts supplier EU Automation, explains why it is time for manufacturers to use big data processes to adapt to this new normal.
A digital future lies ahead. By acting early, being bold and decisive, manufacturers can accelerate their factory digital transformation to mitigate implications of COVID-19. After all, nobody knows how long the disruption caused by the global pandemic will last or what shape the recovery will take. Already, it has forced businesses to adjust to a new digital reality far sooner than they might have planned, and there is no guarantee that, once it is over, things will return to how they were before.
For example, it is generally accepted that the explosion of Chinese e-commerce was a direct result of the severe acute respiratory syndrome (SARS) epidemic in 2003. That was their new normal. And since, China’s annual economic output multiplied more than eightfold, to nearly $14 trillion from $1.7 trillion — that is according to the World Bank.
One route manufacturers can take to adapt to today’s new normal is by investing in artificial intelligence (AI) technologies like big data. Big data is a term that describes the large volume of data that inundates a business on a day-to-day basis, which can help bridge the gap caused by the pandemic’s impact on the workforce.
However, it is not the amount of data that is essential, it is what organizations do with the data that matters. Big data can be analyzed for insights that lead to better decisions and strategic moves. This can only be achieved by remembering the three Vs — volume, velocity and variety.
Volume
The first V, volume, refers to the amount of data handled in big data systems. Big data is large in volume and relies on massive datasets, often as large as petabytes or zetabytes, to operate. To put this in perspective, one petabyte is one million gigabytes, which is the combined storage capacity of 15,625 iPhone 11s. This scale might seem unfathomable, but these large datasets are not as difficult to collate as you might think.
For example, Facebook boasts more users than China has people. Each of those users store a lot of photographs, with Facebook storing roughly 250 billion images. As far back as 2016, Facebook had 2.5 trillion posts, which is a number incredibly hard to envisage.
Taking this theory onto the factory floor, adding more connected sensors to devices means all that telemetry data will add up. In fact, the increasing prominence of smart technology, like smart sensors, means that manufacturers can capture large volumes of data from almost any type of machine.
For instance, variables such as temperature, humidity, pressure, vibration and changes in operations can be used to monitor individual components and predict equipment failure. Data analytics tools can use this mass collection of information to predict when a component is likely to fail, meaning maintenance can be planned upfront, minimizing costly unplanned downtime.
Velocity
The second V, velocity, refers to the speed at which data is generated and the time it takes for this data to be processed. Returning to our Facebook example, users upload more than 900 million photos a day. So, the 250 billion number mentioned, will be outdated in a matter of months.
In short, data does not only need to be acquired quickly, but also processed and used at a faster rate. Add in the fact that the Industrial Internet of Things (IIOT) continues to increase its prevalence in the factory, more connected sensors will be out in the world transmitting data at a near constant rate.
For example, modern smart sensor technology using non-contact, highspeed laser sensors can detect issues traditional accelerometers cannot. With these laser sensors able to rapidly identify everything accelerometers can, as well as monitoring characteristics such as joint domain and modal analysis, condition monitoring capabilities are vastly improved. But, the onus remains of using real-time data to make the most accurate and appropriate decisions.
Variety
Onto the third and final V, variety. This refers to the different types of data involved in big data processes. Equipment status, parts condition, inventory and product service life are just some of the variables that create the complex web of data that must be managed by manufacturers.
Managing this data requires multiple integrated systems to create an all-encompassing view of the facility. For example, parts condition monitoring data might identify when a machine component is showing signs of failure. This can be automatically cross-referenced with the facility’s inventory data to see if a replacement is available.
If replacement parts are unavailable in your facility’s inventory, they can be ordered from an industrial parts supplier in advance to prevent machine failure. With supply chains being heavily disrupted by the pandemic, planning maintenance and ordering replacement parts before downtime occurs is a necessity.
Although COVID-19 is disrupting business on an unprecedented scale, it should encourage manufacturers to use big data processes and adapt to this new normal. By simply remembering the three V’s, manufacturers can analyze big data for insights that lead to better decisions and strategic moves.
www.euautomation.com