www.ptreview.co.uk
23
'22
Written on Modified on
Qualcomm News
CVPR 2022 Pushing the limits in AI research
The Computer Vision and Pattern Recognition (CVPR) conference is one of the most pivotal AI events of the year in all engineering and computer science, gathering thousands of researchers and engineers worldwide to share discoveries, establish collaborations, and push the field forward.
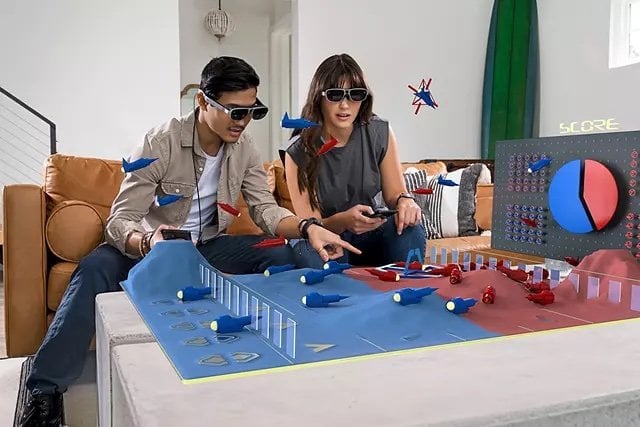
We’re excited to be back at an in-person CVPR for the first time since 2019. Whether you’re attending CVPR or just curious about what Qualcomm AI Research has in store, read on to learn about our latest papers, workshops, demos, and other AI highlights.
Our CVPR papers and poster presentations
At premier conferences like CVPR, meticulously peer-reviewed papers set the new state of the art (SOTA) and contribute impactful research to the rest of the community. We’d like to highlight four of our accepted papers advancing the frontiers in computer vision.
Our paper, “Panoptic, Instance and Semantic Relations (PISR): A Relational Context Encoder to Enhance Panoptic Segmentation,” introduces a novel neural architecture for panoptic segmentation where the goal is to label image pixels into things (countable objects, such as individual cars and pedestrians) and stuff (uncountable concepts like sky and vegetation). This fundamental feature empowers many applications like autonomous driving and augmented reality.
Our framework effectively captures the rich context among stuff and things while automatically focusing on important ones, which was not possible before. PISR is a universal module that can be integrated into any panoptic segmentation method. Our experiments show that PISR achieves superior performance on all benchmarks.
Optical flow estimates how pixels are displaced across two images. It is widely employed for many video and enhancement-analysis applications, from video super-resolution to action recognition. One major challenge for optical flow is insufficient real-world training data with ground-truth annotations. To alleviate this, “Imposing Consistency for Optical Flow Estimation” introduces novel and effective consistency strategies leveraging self-supervised learning while requiring no additional annotations. Our method achieves the best foreground accuracy on benchmarks even though it uses only monocular image inputs.
Inverse rendering is the task of decomposing a scene image into the intrinsic factors of shape, lighting, objects, and materials, thereby enabling downstream tasks like virtual object insertion, material editing, and relighting. This problem is particularly challenging for indoor scenes that exhibit significant appearance variations due to myriad interactions between arbitrarily diverse object shapes, spatially-changing materials, and complex lighting.
“Dense Vision Transformers for Single-Image Inverse Rendering in Indoor Scenes” proposes a new transformer architecture to simultaneously estimate depths, surface normals, albedo, roughness, and lighting from a single image. It delivers high-quality, globally coherent reflectance function, geometry, and lighting prediction. In addition, downstream tasks greatly benefit from our improvements, attaining greater photorealism than in prior works.
Most face relighting methods struggle to handle hard shadows, such as those cast by the nose, since they do not directly leverage the estimated face geometry while synthesizing the image. “Face Relighting with Geometrically Consistent Shadows” proposes a novel differentiable algorithm for synthesizing hard shadows based on ray tracing, which we incorporate into training our face relighting model. Our method produces more geometrically consistent shadows than previous face relighting methods while also achieving SOTA face relighting performance under directional lighting.
Design choices of common semantic segmentation architectures often rely on operations that are inefficient on current hardware, and their complexity impedes the use of model acceleration tools. Our Efficient Deep Learning for Computer Vision (ECV) Workshop paper "Simple and Efficient Architectures for Semantic Segmentation" provides a family of extremely competitive encoder-decoder architectures that extend across a variety of devices, ranging from highly capable desktop GPUs to power limited mobile platforms, enabling effective semantic segmentation baselines for computer vision practitioners. We offer the model definitions and pretrained weights at https://github.com/Qualcomm-AI-research/FFNet.
Workshops at CVPR
Wireless AI Perception (WAIP)
Using radio frequencies for perception as a new type of camera facilitates inventing an entirely new generation of solutions that use wireless signals to their full potential for widespread applications. Qualcomm AI Research is honored to be co-organizing the very first Wireless AI Perception Workshop (June 20) to highlight cutting-edge approaches and recent progress in the growing field of joint wireless perception using machine learning and possibly also other modalities, such as images and 3D data. We look forward to researchers and companies in wireless perception presenting their progress and discussing novel ideas that will shape the future of this area.
Omnidirectional Computer Vision (OmniCV)
Omnidirectional cameras are already widespread in many applications such as automotive, surveillance, photography, and augmented/virtual reality that benefit from a large field of view. Qualcomm AI Research is co-organizing the Omnidirectional Computer Vision Workshop (June 20) to bridge the gap between the research and real-life use of omnidirectional vision technologies. This workshop links the formative research that supports these advances and the realization of commercial products that leverage this technology. It encourages development of new algorithms and applications for this imaging modality that will continue to drive future progress.
Our CVPR demos
To show that our AI and CV research topics are more than just theory and applicable in the real world, we bring them to life through demonstrations. We hope that you can stop by our booth #1401 to see them live. Here are a few demos that I’d like to highlight.
Temporally consistent video semantic segmentation on a mobile device
Existing segmentation methods often have flickering artifacts and lack temporal consistency between video frames. They often rely on motion estimation for regularization. However, optical flow is not always available and reliable. Besides that, it is expensive to compute. In this demo, we showcase consistent and robust video segmentation using on-device adaptation running in real time on a smartphone. AuxAdapt, our novel online adaptation method, helps enhance the temporal consistency of the original output.
To enable real-time adaptation on a smartphone, we utilize heterogeneous computing to distribute AI tasks to the DSP and GPU, efficiently accelerating AI computations. For the video frames without AuxAdapt, you can see flickering in the base network output. With AuxAdapt, no flickering occurs. Our optimizations reduce the flickering significantly and decrease inference time by over 5x to 37 milliseconds and achieve real-time performance. AuxAdapt is an accepted CVPR demo, so if you are at the conference, stop by the demo area at 10 a.m., from Tuesday to Thursday.
Advanced CV capabilities enabling diverse use cases
Our research teams are working on virtually every CV technology, from segmentation, object recognition, and super-resolution to computational camera and 3D reconstruction. These core CV technologies are enhancing experiences on smartphones, automotive, XR, and IoT. For example, we are demonstrating panoptic segmentation, semantic object tracking, super resolution, CV for smart campuses, photorealistic facial avatars, and a skin temperature screening solution.
For example, our 4K super-resolution demo runs faster than real-time on-device at over 100 frames per second, enabling more immersive gaming and XR experiences. In addition, our real-time semantic object tracking demo showcases accurate one-shot long-term tracking of any object of interest, enabling camera features like auto-zoom for smooth pan, tile, and zoom control.
Enabling technologies powering the metaverse
We believe that XR is going to be the next mobile computing platform. Our foundational XR research is addressing the fundamental challenges of making XR a mass market device. Our booth demos showcase a live VR demo on a commercial device, our AR reference design running a Snapdragon Spaces application, and fundamental CV technology advancements in 6 degrees-of-freedom tracking, 3D reconstruction, and much more.
AIMET Model Zoo
We are also very excited to share that the Qualcomm Innovation Center (QuIC) has released several image super-resolution models with different architecture types to the AI Model Efficiency Toolkit (AIMET) . AIMET provides the recipe for quantizing popular 32-bit floating point (FP32) models to 8-bit integer (INT8) models with little loss in accuracy. The tested and verified recipes include a script that optimizes TensorFlow or PyTorch models.
We hope that you can take advantage of these new super-resolution models since this CV capability is very useful across many applications, from gaming and photography to XR and autonomous driving. With our quantization optimizations, super-resolution models will run faster and much more efficiently on INT8 hardware while retaining FP32-like image quality, expanding the possibilities for additional use cases and different form factors.
We hope to meet you at CVPR or future AI conferences and share our impact on AI innovation. At Qualcomm Technologies, we make breakthroughs in fundamental research and scale them across devices and industries. Our AI solutions are powering the connected intelligent edge. Qualcomm AI Research works together with the rest of the company to integrate the latest AI developments and technology into our products — shortening the time between research in the lab and delivering advances in AI that enrich lives.
www.qualcomm.com